SRBench: Symbolic Regression Benchmarks
The methods for symbolic regression (SR) have come a long way since the days of Koza-style genetic programming (GP). Our goal with this project is create a living benchmark of modern symbolic regression methods, in the context of state-of-the-art ML methods, and with a view towards high-impact applications in the health sciences.
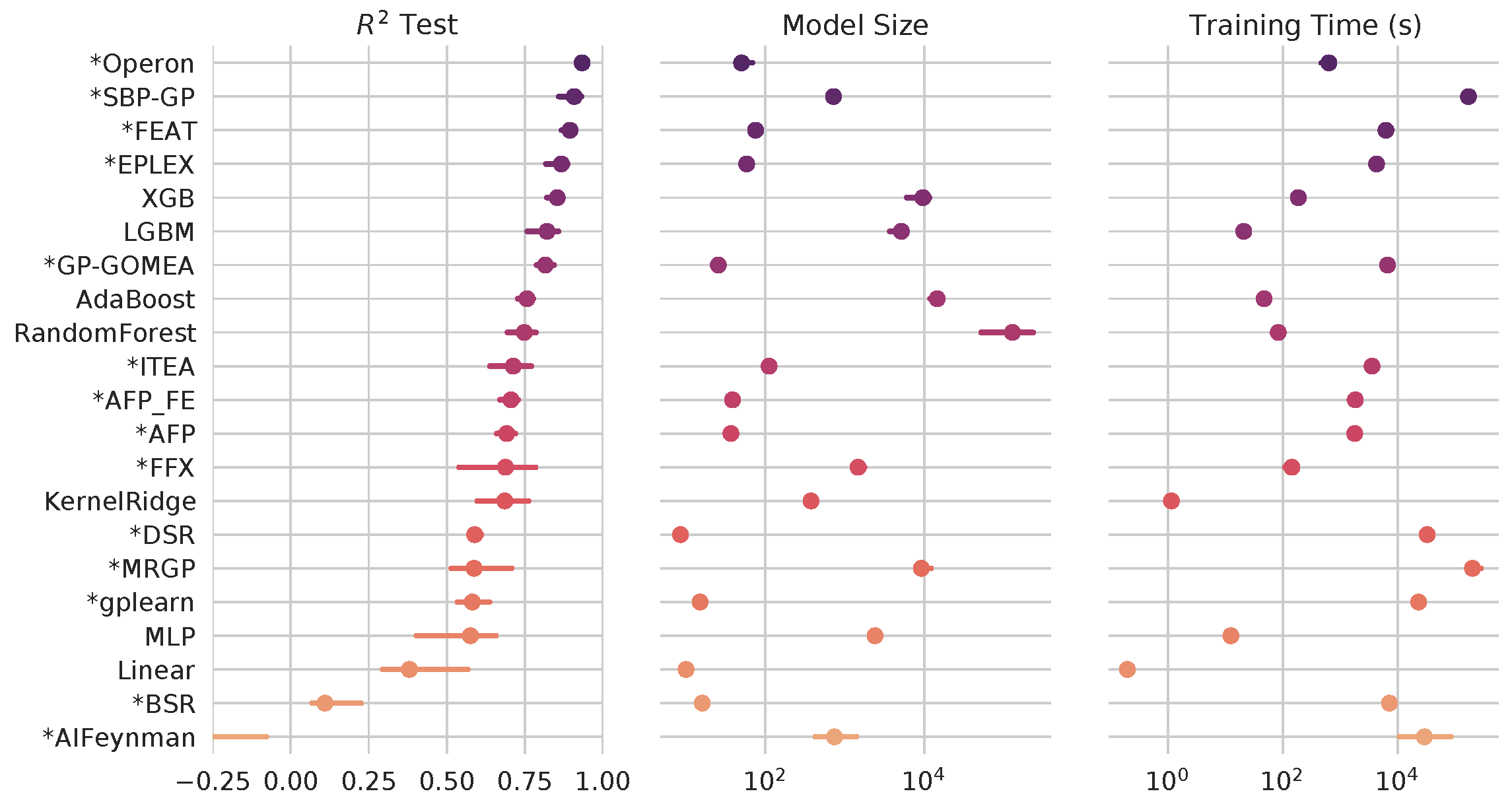
Code
Selected Papers
SRBench++: Principled Benchmarking of Symbolic Regression With Domain-Expert Interpretation
IEEE Transactions on Evolutionary Computation
Contemporary Symbolic Regression Methods and their Relative Performance
Proceedings of the Neural Information Processing Systems Track on Datasets and Benchmarks
A comparative study of GP-based and state-of-the-art classifiers on a synthetic machine learning benchmark
Proceedings of the Genetic and Evolutionary Computation Conference Companion
Where are we now? A large benchmark study of recent symbolic regression methods
Proceedings of the 2018 Genetic and Evolutionary Computation Conference