A new perspective on how this social theory relates to fair machine learning.
Reducing health disparities in clinical decision support with fair machine learning
We are developing machine learning algorithms that can adapt to changing hospital environments in real time and make predictions that are equally accuracy among patient subpopulations. We are studying these algorithms for patient admission risk predictions in emergency rooms.
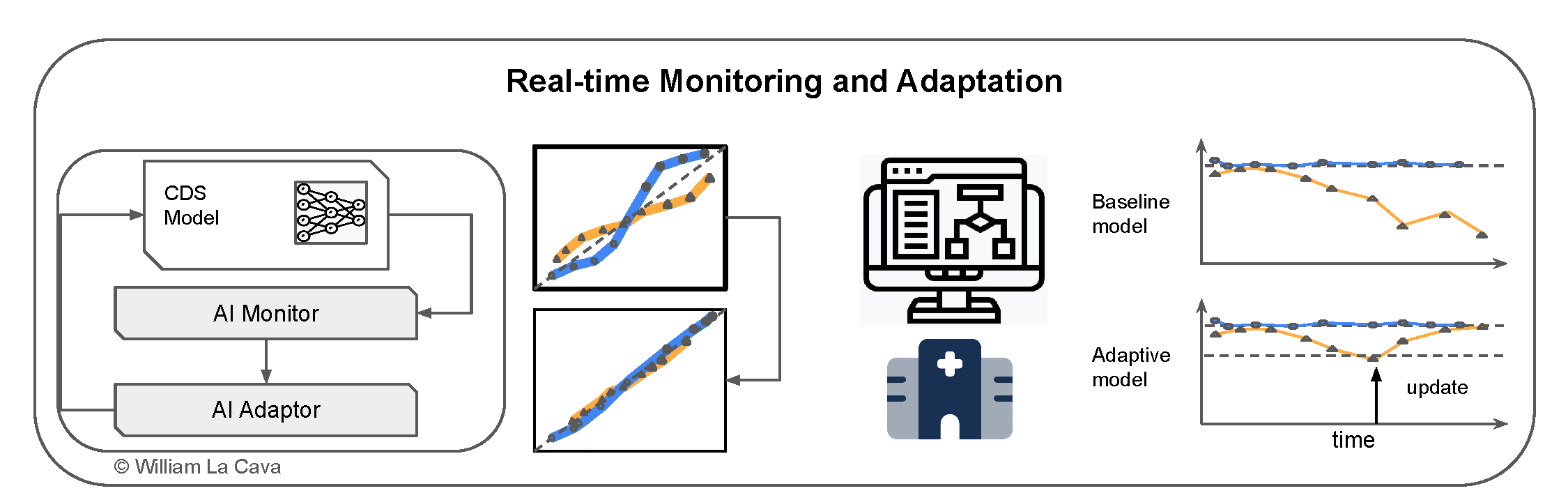
Code
Press
🎉 La Cava and Lett’s fair ML tool, Interfair, won first place in the 2023 NIH Challenge, Bias Detection Tools for Clinical Decision Making.
Related Posts
Selected Papers
Fair admission risk prediction with proportional multicalibration
Proceedings of the Conference on Health, Inference, and Learning