FOMO: Fairness-Oriented Multi-objective Optimization
Improving the fairness of machine learning models is a nuanced task that requires decision makers to reason about multiple, conflicting criteria. The majority of fair machine learning methods transform the error-fairness trade-off into a single objective problem with a parameter controlling the relative importance of error versus fairness. Our lab takes a different approach, developing flexible optimizers that characterize the error-fairness tradeoff surface by integrating multi-objective optimization into existing machine learning models.
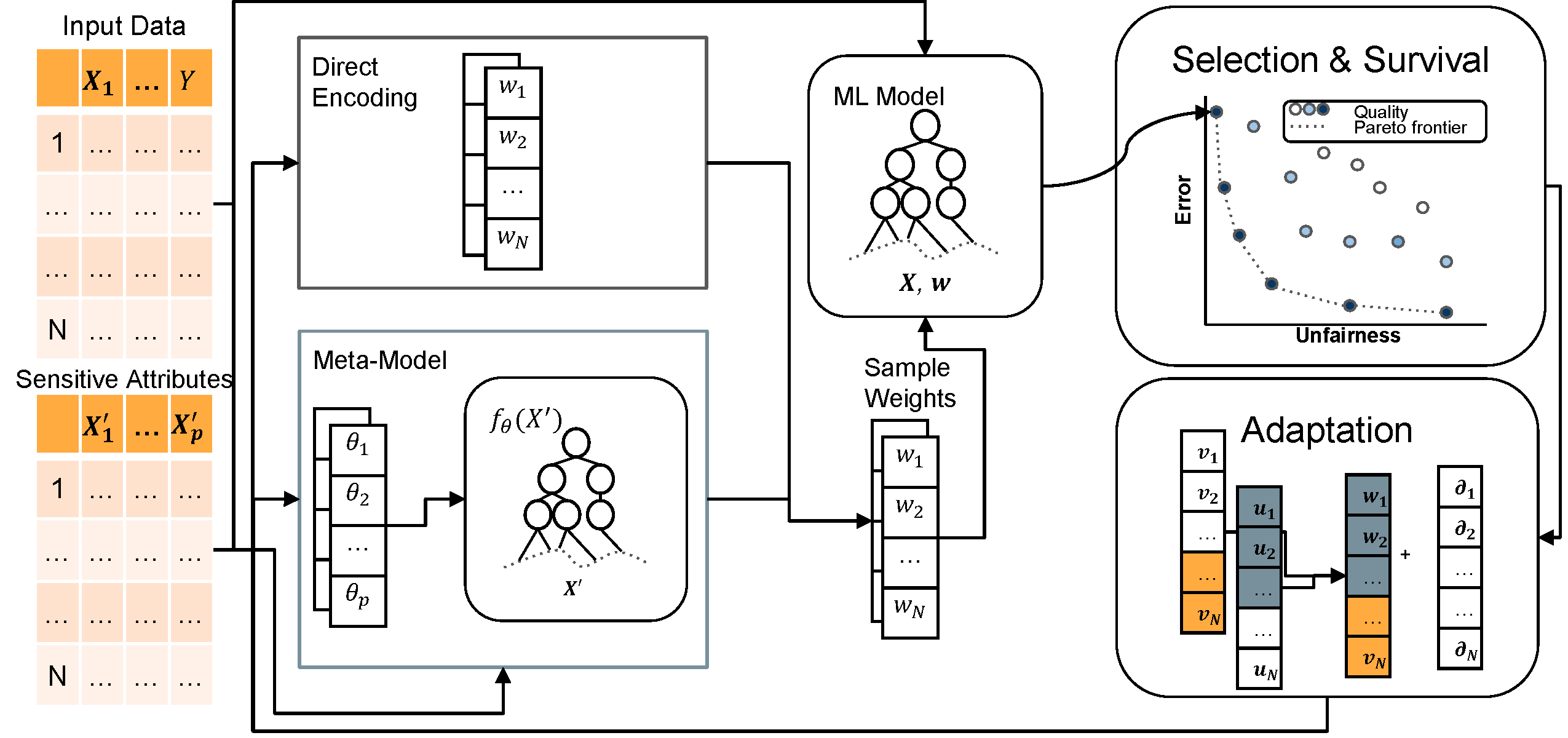
Code
Selected Papers
Optimizing fairness tradeoffs in machine learning with multiobjective meta-models
Proceedings of the 2023 Genetic and Evolutionary Computation Conference (GECCO)
Genetic programming approaches to learning fair classifiers
Proceedings of the 2020 Genetic and Evolutionary Computation Conference