AI-EFM: Predicting Fetal Distress During Labor
Electronic fetal monitoring (EFM) is currently used in the vast majority of all hospital births in the United States to monitor the fetal heart rate. Despite its ubiquity, substantial limitations persist in the efficacy, reliability, and accuracy of EFM in accomplishing its primary intended goal of preventing intrapartum fetal injury. One of the greatest challenges that obstetricians face is interpreting EFM; how to distinguish fetal distress that warrants an emergency cesarean delivery (CD) from a false alarm that can safely be ignored. The goal of this project is to develop and assess AI algorithms that predict the need for interventions during birth using electronic fetal monitoring (EFM) data.
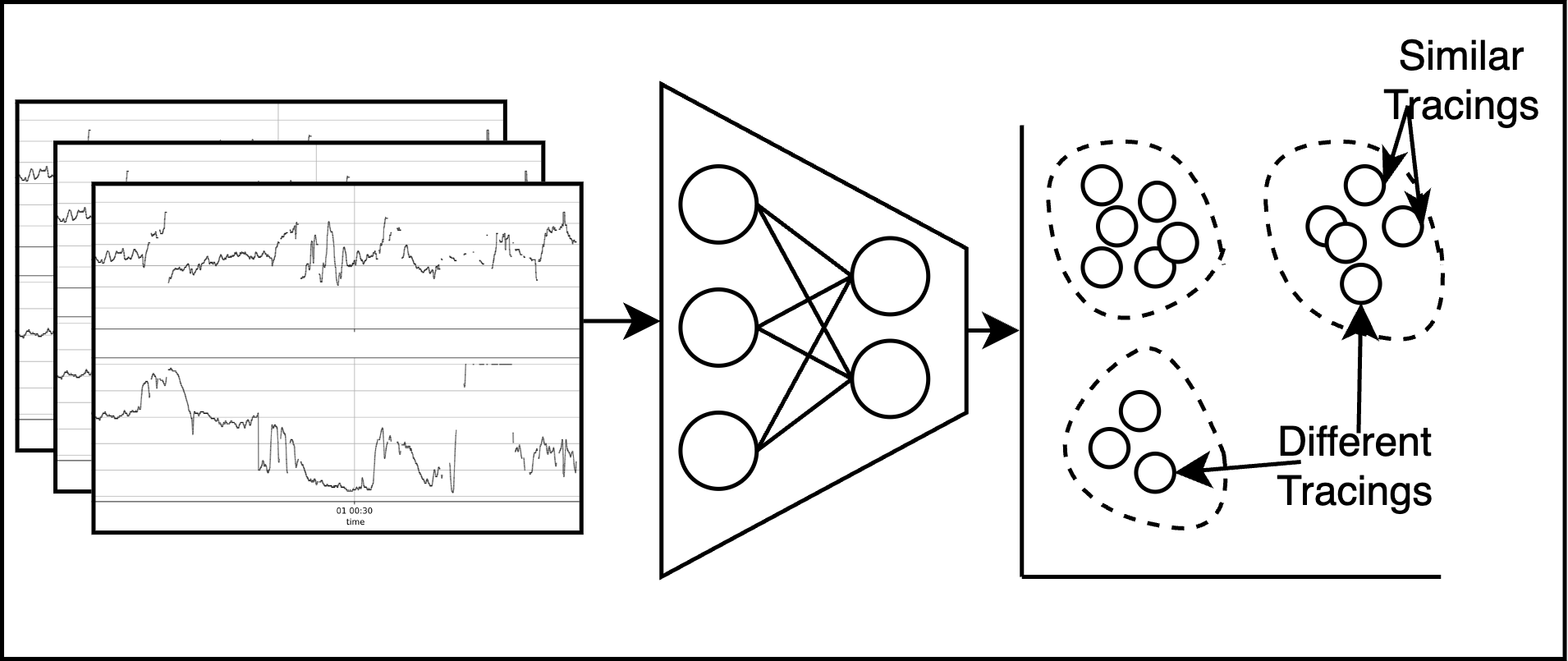
Selected Papers
Intrapartum electronic fetal heart rate monitoring to predict acidemia at birth with the use of deep learning
American Journal of Obstetrics and Gynecology
Accuracy of deep learning models in interpreting intrapartum fetal monitoring to predict fetal acidemia
American Journal of Obstetrics and Gynecology